Project details
Employer
Bicycle AI
Role
UX Designer, Lead Front-end Engineer
Category
Featured, Platform Design, UX
Overview
BicycleAI is a yCombinator company from their 2017 batch. The company was trying to build AI tools that would work on top of existing online customer care solutions and provide response automation with a human-in-the-loop system. These tools were built with the objective of easing the customer care agent’s work flow and increasing query throughput with automated chat handling, forwarding issues to human agents only in cases that require human attention.
I joined the company in late 2015, as the User Experience Designer and Lead Front-end Engineer.
High level goals
○ Develop an understanding of the nuances of the customer-care conversation
○ Identify points of leverage where AI can assist human operators
○ Aid the research and development of said AI system with quick design probes and prototypes
○ Build a scalable platform that provides all the required functionality to run de-centralized customer care operations.
Research
The research process included:
○ Co-design phase with partner businesses
○ Rough digital prototypes and probes to understand the expectations from an AI customer care system
○ Collaboration with a team of experienced customer care agents, who served as AI trainers and as experts from the agent side of the picture.
The major research insights from this project were:
○ For most customer care conversations on a website chat, customers prefer to the point functional responses to their queries, as opposed to a more ‘human’ conversation
○ For complex queries, customers do prefer to have a human level response
○ Lack of cultural context heavily impedes our ability to set up customer service as a distributed global network of individuals providing a service. The AI tools needed to help bridge that gap
○ A large amount of customer service queries coming in to most organizations, can be served by existing faq’s, but in the absence of easily discover-able information, customers resort to talking to a ‘human’.
Outcome
To address these concerns we built a pipeline of technology for our business customers:
○ A method to parse all existing customer care conversations into a usable knowledge base
○ Provide a platform for businesses to mobilize these knowledge bases in the form of a FAQ interface, powered by our Natural Language Processing technology
○ Using the same technology, provide tools for customer care agents, to respond to common queries, while continuing to use existing systems.
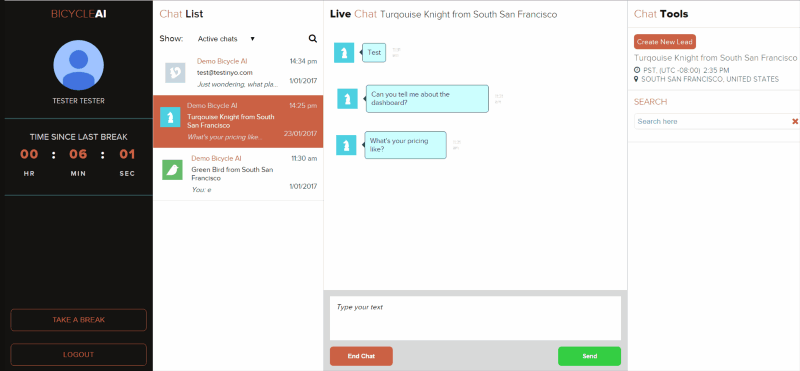
To ease the work flow of a typical customer care agent, with the help of the Machine Learning team, we were able to design a system that could automatically pick up questions from a customer, and provide pre-tailored answers for the agent to choose from. The agent could also choose to edit, or craft a custom response, which the system would then feed back into it’s knowledge base, and make available for future queries.
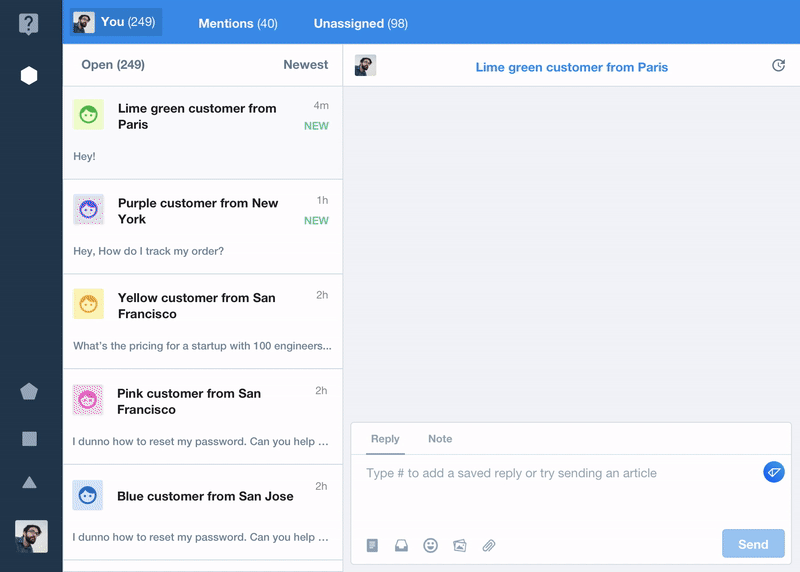